In a recent interview with Yahoo Finance, Richard Windsor, Founder and CEO of Radio Free Mobile, delivered a thought-provoking statement that has sparked discussions within the field of Artificial Intelligence. Windsor claimed that the current state of AI falls far short of the forthcoming age of super AI information computing, citing a crucial deficit in causal understanding within contemporary AI algorithms as a primary reason for this gap. Let's look at the significance of the causal understanding in AI and the challenges we may need to overcome as we advance toward the era of super AI.
The rise of Artificial Intelligence has made remarkable strides in recent years, permeating various facets of our lives. From virtual assistants like Siri and Alexa to autonomous vehicles and predictive analytics, AI has evolved from a futuristic concept into an integral part of our daily routines. However, as Windsor highlights, there is still a long road ahead before we reach the stage of super AI, capable of processing information and making decisions at a level that rivals or surpasses human intelligence. Windsor's assertion centers around the concept of causal understanding, a critical aspect of human cognition that is currently lacking in AI systems. Causal understanding refers to the ability to comprehend the cause-and-effect relationships that govern the world around us. Humans possess an innate capacity to recognize that certain actions lead to specific consequences, and this understanding forms the basis of our decision-making processes. In contrast, most AI algorithms rely on statistical correlations rather than true causal understanding. They can identify patterns and associations within vast datasets but struggle to discern the underlying mechanisms driving these patterns. This limitation is particularly evident in scenarios where causal relationships are complex or non-linear.
Causal understanding is not merely an abstract concept; it has profound implications for AI's ability to operate effectively and ethically in complex environments. Consider, for instance, the application of AI in healthcare. An AI system that lacks causal understanding might identify a statistical correlation between a specific symptom and a particular disease, but without comprehending the underlying causes, it may suggest incorrect treatments or misdiagnose patients. Similarly, in autonomous vehicles, an AI system that relies solely on correlations may struggle to make nuanced decisions in unpredictable traffic scenarios. It may recognize that certain patterns of vehicle behavior precede accidents but may not understand the causal factors behind those patterns, limiting its ability to adapt to new situations effectively.
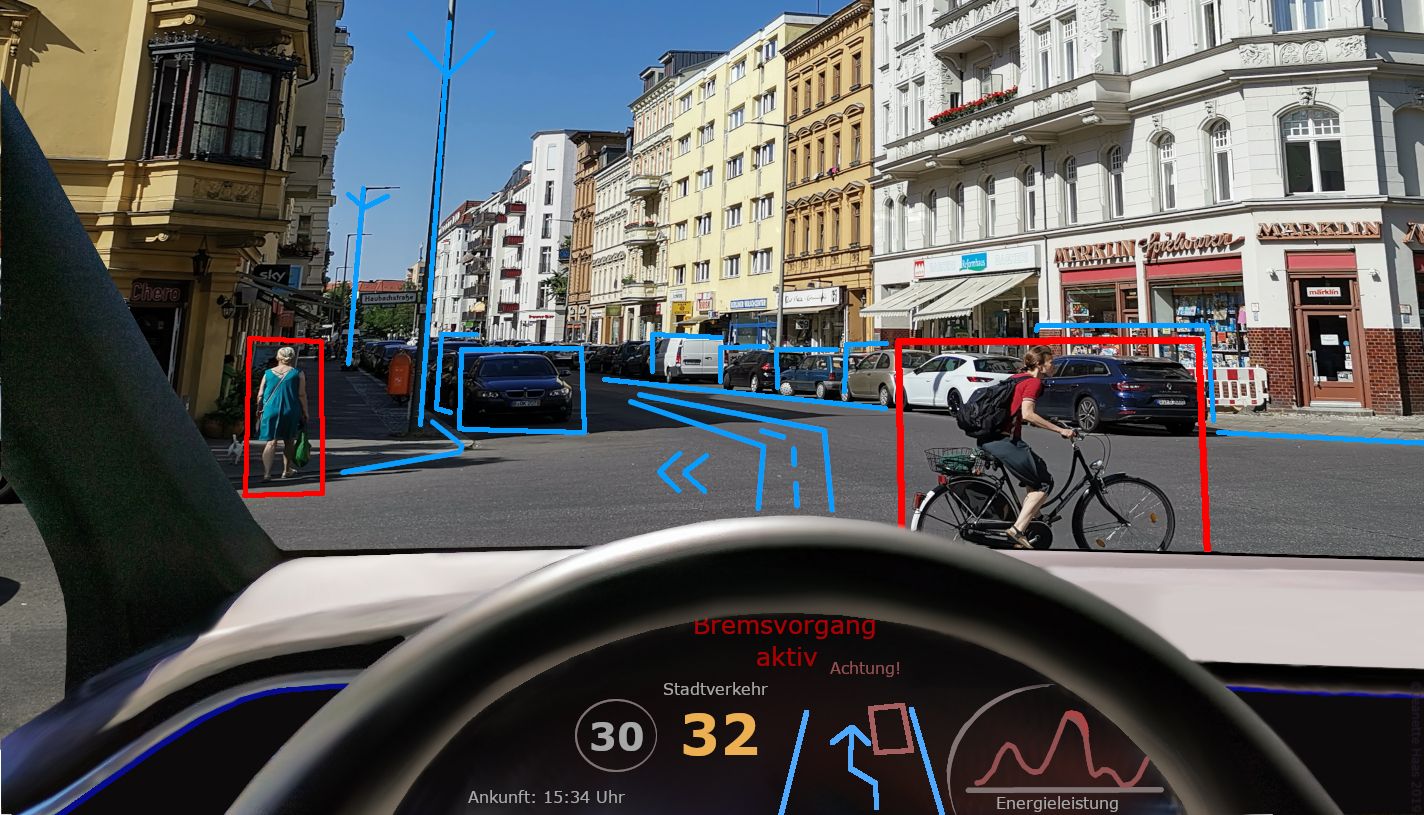
To bridge the gap between current AI capabilities and the vision of super AI, researchers and engineers must tackle the challenge of causal understanding head-on. Here are some key areas of focus:
1. Causal Inference Algorithms: Developing AI algorithms that can infer causal relationships from observational data is a critical step. These algorithms should go beyond identifying correlations and aim to uncover the underlying causal mechanisms.
2. Human-Machine Collaboration: Combining the strengths of AI with human expertise can accelerate progress. Human intuition and causal reasoning can complement AI's analytical capabilities, helping to fill the gaps in understanding.
3. Interdisciplinary Research: Collaboration between experts from various fields, including computer science, neuroscience, and philosophy, can provide fresh perspectives on the problem of causal understanding in AI.
4. Ethical Considerations: As AI systems become more sophisticated, ethical questions surrounding their decision-making processes become increasingly important. Ensuring that AI understands causality can help mitigate the risks of biased or unfair decision-making.
5. Continuous Learning: AI systems should be designed with the ability to learn and adapt their causal models over time. This adaptability is essential for handling dynamic and evolving environments.
Windsor's assertion that the current state of AI falls short of super AI due to the lack of causal understanding within AI algorithms points to numerous implications for the future of AI. Causal understanding is indeed a critical component of human intelligence, enabling us to make sense of the world and make informed decisions. While we have made remarkable progress in AI, we must acknowledge the limitations of current systems and actively work to address the deficit in causal understanding. As we continue to advance in this direction, we can hope to see AI systems that not only excel in recognizing patterns but also understand the deeper relationships that govern our world. In doing so, we pave the way for a future where AI operates at the level of superintelligence, enhancing our lives in ways we can scarcely imagine today.