Creative artificial intelligence (AI) has the potential to impact (dare we even say transform) the way we approach art, design, and storytelling. From AI-generated music and paintings to algorithm-driven filmmaking and literature, the potential appears to be rather vast. However, as this technology becomes more integrated in creative industries and creative economies at large, fundamental questions about bias and its long-term effects on creativity, diversity, and integrity arise. Can AI democratize creative practices, or will it embed systemic limitations that constrain innovation? Can AI be used as a tool that fosters experimentation and exploration, leading to emergent forms of creative practice?
Algorithmic bias can emerge through the personal biases of the engineers who design and train algorithms or through systematic errors within AI systems. In either case, algorithmic bias can have a disproportionate effect and detrimental impact on certain groups or ideas. In creative AI, these biases manifest in ways that can homogenize cultural expression, reinforce stereotypes, and further marginalize underrepresented and critically important voices. At its core, these issues stem from the datasets used to train AI models and the processes by which these models generate outputs.
Training Data in Reinforcing Bias
Creative AI models often rely on datasets that reflect dominant cultural narratives and aesthetics, typically skewed toward Western or more “mainstream” creative practices. This reliance can result in outputs that reinforce existing hierarchies, overshadowing diverse or non-Western creative forms. Timnit Gebru and Dr. Joy Buolamwini, pioneers in AI ethics, have highlighted how AI training datasets frequently reflect and amplify historical biases. Gebru’s research on dataset transparency and Buolamwini’s work on facial recognition biases have revealed that AI models disproportionately underperform when processing data related to underrepresented groups. This phenomenon extends to creative AI, where these biases shape artistic outputs, often excluding marginalized voices and perspectives.
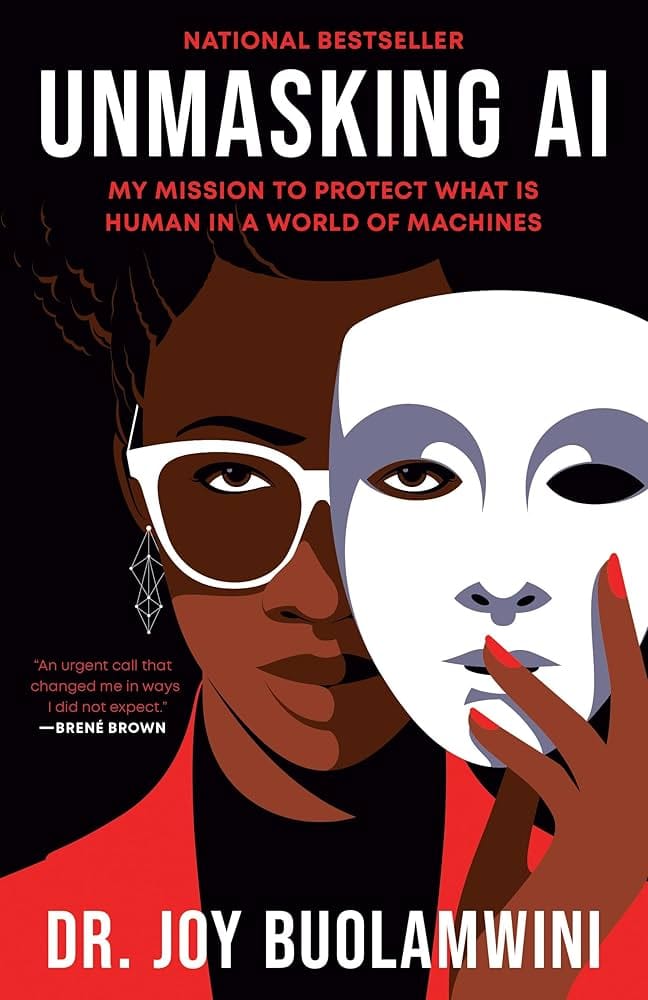
For instance, generative models like OpenAI's DALL-E and MidJourney produce imagery that often aligns with popular design trends in Western cultures, making it harder for underrepresented cultures to see authentic representation in AI-generated works. Buolamwini’s work in algorithmic auditing underscores the necessity for regular evaluation of AI-generated media to prevent further systemic exclusion.
Training datasets frequently include biased representations of gender, race, and social roles. These biases seep into creative outputs, perpetuating stereotypes and limiting how certain groups are portrayed. Gebru has warned about the risks of AI reinforcing historical power imbalances in media and design. For example, AI systems generating visual art or storytelling may depict women in traditional caregiving roles or assign stereotypical attributes to minoritized populations, amplifying harmful tropes. Without intervention, these biases can further entrench societal prejudices rather than challenge or diversify them.
Intellectual Property and Ethical Considerations
AI-generated creative outputs raise questions about intellectual property and the attribution of creative credit. Many AI systems are trained on creative works by humans, often without their knowledge, consent, or compensation. This practice risks devaluing the labor and cultural heritage embedded in those works, particularly for marginalized communities whose contributions have historically been overlooked.
Gebru’s advocacy for ethical AI development includes calls for increased transparency in how training data is sourced and used. Ensuring that artists and designers have control over how their works are incorporated into AI models is essential to preserving creative integrity.
Algorithmic Feedback Loops
One of the most concerning aspects of algorithmic bias in creative AI is arguably its potential to create feedback loops. When AI-generated works enter the creative ecosystem, they influence future datasets, compounding existing biases. Gebru’s research on dataset curation warns that these self-reinforcing cycles can deepen existing disparities in representation.
For example, if AI tools prioritize certain art styles or themes due to their prevalence in initial training data, these preferences will become even more dominant over time, further marginalizing alternative voices. Additionally, personalized recommendation algorithms in platforms like Spotify or YouTube contribute to echo chambers, where users are exposed to increasingly narrow subsets of creative content. This can potentially impede the discovery of diverse, experimental, or emergent works and reinforce mainstream tastes.
Addressing Bias
As creative AI continues to evolve, addressing algorithmic bias requires a multifaceted approach. Ethical guidelines, technical innovations, and community-driven initiatives are essential to fostering fairness and diversity. The foundation of any AI system is its dataset. To counteract bias, developers must curate datasets that reflect a wide array of cultures, identities, and creative practices. Buolamwini’s work with the Algorithmic Justice League advocates for dataset audits and the inclusion of historically marginalized groups in AI training data.
In her book Atlas of AI, Kate Crawford stresses the need for transparency in dataset curation, emphasizing that ethical AI development begins with accountability in data sourcing. Similarly, organizations like the Partnership on AI advocate for creating datasets that align with values of inclusivity and fairness. Engaging artists and cultural practitioners from marginalized communities in the design and training of AI systems is crucial. Participatory models enable local communities to shape AI systems that reflect their unique values and aesthetics. For instance, collaborative initiatives in indigenous art preservation are exploring how AI can amplify rather than exploit cultural heritage.
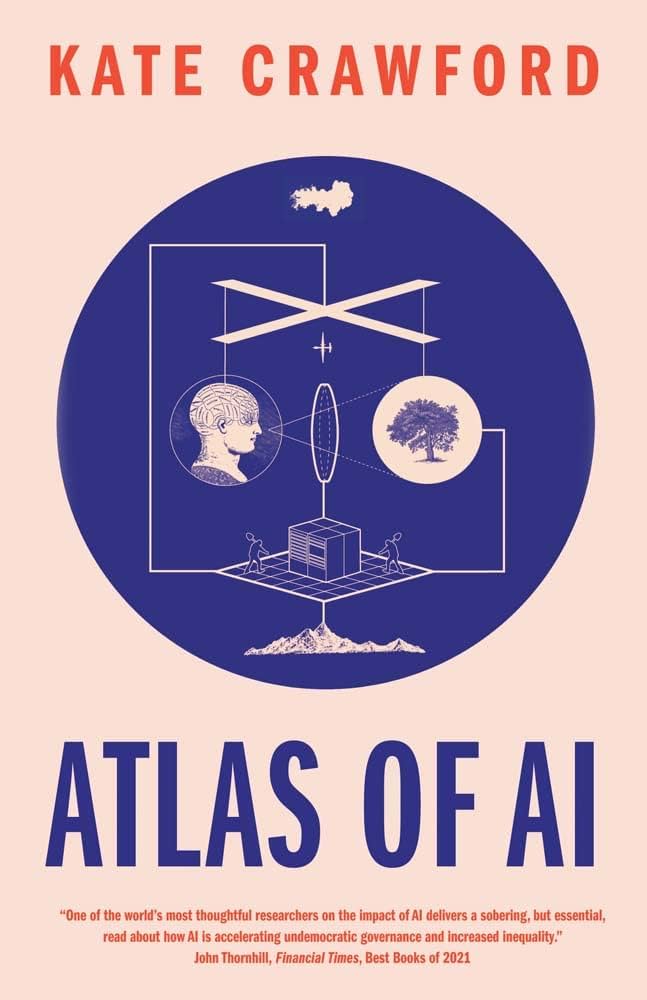
Policy and Regulation
Governments and industry bodies play a critical role in addressing algorithmic bias. Policy frameworks are needed to regulate the use of creative AI, ensuring transparency, accountability, and fair compensation for contributors. This includes:
- Establishing clear guidelines for how AI can use copyrighted works in training datasets.
- Allowing users to modify AI-generated outputs that misrepresent or harm cultural identities.
- Developing industry-wide standards for bias audits and diversity metrics.
Despite these challenges, creative AI has the potential to democratize creativity and amplify underrepresented voices. Open-source initiatives and decentralized AI systems offer pathways for more equitable innovation. By sharing tools and datasets transparently, these approaches allow diverse stakeholders to scrutinize and improve AI systems.
Hybrid human-AI collaborations also hold promise. When humans retain control over creative decisions and processes, they can use AI as a tool within their practice rather than as a gatekeeper.
Rethinking the Ethics of Creative AI
Algorithmic bias in creative AI is not just a technical problem; it is a cultural and ethical challenge that requires collective action. By addressing biases in datasets, fostering transparency, and ensuring community participation, we can build AI systems that support creative processes rather than replace them. The insights of Gebru and Buolamwini provide essential frameworks for recognizing and mitigating bias, ensuring that creative AI serves as a tool for diverse artistic expression rather than a mechanism of exclusion.